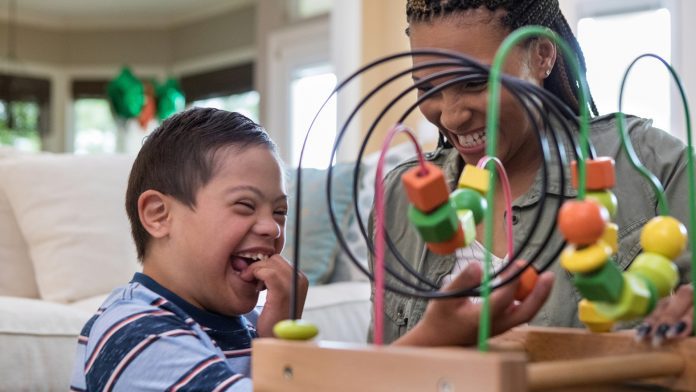
A novel machine learning technology has demonstrated impressive efficiency in detecting genetic syndromes in children, a significant advancement in diagnosing a plethora of disorders.
In a study led by the Children’s National Hospital, researchers developed a machine learning tool that achieves rapid screening of genetic syndromes with an average accuracy of 88%, recommending further investigation or referral to a specialist. The groundbreaking machine learning technology has been trained with data from 2,800 paediatric patients from 28 countries and considers the face variability related to age, sex, and racial and ethnic background.
Marius George Linguraru, the senior author of the study and principal investigator in the Sheikh Zayed Institute for Pediatric Surgical Innovation at Children’s National Hospital, said: “We built a software device to increase access to care and a machine learning technology to identify the disease patterns not immediately obvious to the human eye or intuition and to help physicians non-specialised in genetics. This technological innovation can help children without access to specialised clinics, which are unavailable in most of the world. Ultimately, it can help reduce health inequality in under-resourced societies.”
The study is published in The Lancet Digital Health.
Identifying genetic syndromes
The pioneering machine learning technology utilises a facial photograph of the patient to ascertain the presence of any genetic syndromes, with the image being obtained at points-of-care, such as paediatrician offices, maternity wards, and general practitioner clinics.
Marshall L. Summar, the Director of the Rare Disease Institute at Children’s National Hospital, said: “Unlike other technologies, the strength of this program is distinguishing ‘normal’ from ‘not-normal,’ which makes it an effective screening tool in the hands of community caregivers. This can substantially accelerate the time to diagnosis by providing a robust indicator for patients that need further workup. This first step is often the greatest barrier to moving towards a diagnosis. Once a patient is in the workup system, then the likelihood of diagnosis (by many means) is significantly increased.”
Around the world, millions of children are born each year with genetic syndromes, such as Down Syndrome, Williams-Beuren syndrome, and Noonan syndrome. Down syndrome is a condition in which a child is born with developmental delays and disabilities due to having an extra copy of their 21st chromosome. Williams-Beuren syndrome is triggered by a submicroscopic deletion from a region of chromosome seven and is a rare multisystem condition, whereas Noonan syndrome is a genetic disorder caused by a faulty gene that interrupts normal development in multiple parts of the body.
Sadly, the vast majority of children who are born with genetic syndromes live in parts of the world with limited resources and access to genetic screening services, which can be extremely expensive. Additionally, there is also a lack of specialists in these regions who can identify genetic syndromes in early development stages where preventative care is vital to saving children’s lives. This is observed mainly in areas of low-income, limited resources, and isolated communities.
Linguraru said: “The presented technology can assist paediatricians, neonatologists and family physicians in the routine or remote evaluation of paediatric patients, especially in areas with limited access to specialised care. Our technology may be a step forward for the democratisation of health resources for genetic screening.”
Refining the machine learning technique
To optimise their machine learning technology, the team trained the system with 2,800 retrospective facial photographs of children who either had or didn’t have genetic syndromes from 28 countries, including Argentina, Australia, Brazil, China, France, Morocco, Nigeria, Paraguay, Thailand and the US. The architecture of the machine learning technology was designed to account for the normal face variations among populations of diverse demographic groups.
“Facial appearance is influenced by the race and ethnicity of the patients. The large variety of conditions and the diversity of populations are impacting the early identification of these conditions due to the lack of data that can serve as a point of reference,” said Linguraru. “Racial and ethnic disparities still exist in genetic syndrome survival even in some of the most common and best-studied conditions.”
Moving forward, the researchers are aiming to train the technology with more datasets of underrepresented groups, adapting the model to localise phenotypical variations within more specific demographic groups. Furthermore, as well as being an accessible tool for telehealth services to examine genetic risk, there is a range of further applications for the machine learning technology.
“I am also excited about the potential of the technology in newborn screening,” commented Linguraru. “There are approximately 140 million newborns every year worldwide of which eight million are born with a serious congenital disability of genetic or partially genetic origin, many of which are discovered late.”
Children’s National recently announced that it has entered into a licensing agreement with MGeneRx Inc. for its patented paediatric medical device technology.
Nasser Hassan, the acting chief executive officer of MGeneRx Inc., said: “The social impact of this technology cannot be underestimated. We are excited about this licensing agreement with Children’s National Hospital and the opportunity to enhance this technology and expand its application to populations where precision medicine and the earliest possible interventions are sorely needed in order to save and improve children’s lives.”